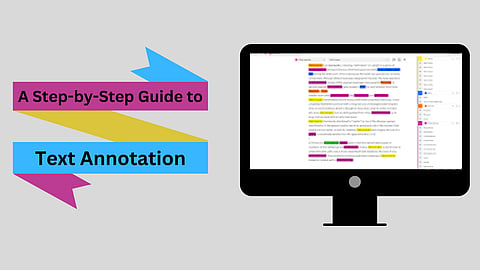
When annotation occurs for textual data present in either text files or images/videos, it gets called text annotation. (Brown Walsh)
By Brown Walsh
An Artificial Intelligence system is an algorithm that learns things about its tasks similar to humans. As much as a marvel it is, there is one critical flaw in it: it is a machine and doesn’t have the instinct to learn like humans. Thus, training is imparted to such algorithms utilizing data annotation by expert developers.
Depending on the application, various forms of training data are applied to the process, with the most notable being images and videos as a part of Computer Vision training. But there is also textual data in the world that the AI should manage, and that is handled by text annotation. With text annotation, your company can leverage the power of AI and build state-of-the-art solutions that can contribute to your company’s growth and data management efforts simultaneously. And it is very useful in present times when conversational AI is all the rage (as evident from the widespread popularity of solutions like ChatGPT).
Your company can and should take advantage of AI that interprets textual data. Through this quick guide, you can learn about how that’s possible with text annotation (via an in-house team or external text annotation service providers).
Data annotation for machine learning involves the tagging of specific data points in a given data sample for the algorithm to discern from the rest of the data in that sample. This is done via various annotation techniques that apply to each data type. When annotation occurs for textual data present in either text files or images/videos, it gets called text annotation.
The tags added to the specific data points are considered metadata that describes those data points’ characteristics. The algorithm being trained relies on this metadata to recognize and isolate those data points, which, after numerous iterations, can do the same in unmarked, input, raw data samples.
You can avail of text annotation services to convert your raw enterprise text data into a dataset that can be used to develop AI/ML models for Natural Language Processing and Computer Vision Applications at your company.
Text is used by companies for various purposes, with each scenario demanding a different approach to using language(s), tome, subject matter, and other parameters. The AI used for these should be able to adapt accordingly, which is why there are many techniques used to develop a variety of AI models for text data.
Entity Annotation
This type of text annotation is used to locate, extract, and demarcate different entities in a data sample. The sample is analyzed for the intended text snippets, which, when found, are highlighted and labeled with tags from a predefined set. This type is used in combination with the Entity Linking type for enhanced outputs.
Entity annotation has the following subcategories:
Named Entity Recognition
Proper names are used to annotate entities here.
Keyphrase Tagging
Involves the accurate location and labeling of keywords and key phrases.
Part-of-Speech Tagging
The function elements of speech, like adjectives, nouns, pronouns, etc., are identified and annotated.
Entity Linking
Applied to extensive data repositories when there’s a need to link entities classified during Entry annotation. URLs connect the labeled entities, providing greater insights into them. Entity linking comes in the following two types:
Disambiguation
Links named entities to knowledge bases containing information about those entities.
End-To-End
Analysis and annotation are together applied to the data here. Also called entity recognition and gets done along with disambiguation.
Text Classification
Also named document classification and text categorization, this technique has annotators analyzing data sets to discern their various qualities and classify the same. It is best used to attach a label to an entire body of text data.
Text classification comes in the following forms:
Document Classification
Documents are classified into various categories to sort and recall them easily.
Product Categorization
It is used to sort products into various categories based on intuitive classes and improve search results. A predetermined set is used to choose the right category.
Sentiment Annotation
It helps recognize the sentiment, emotions, and opinions that appear in a given data sample and label the respective segment accordingly. It helps detect those qualities in large data sets easily.
Linguistics Annotation
It is also called Corpus Annotation and is used to tag language in textual data. Professionals identify and flag the phonetic, semantic, and grammatical components of the data. It is primarily done for NLP applications and has four subcategories:
Semantic Annotation
It consists of annotating word definitions.
Discourse Annotation
It is where anaphors and metaphors are associated with respective antecedent and postcedent subjects
Phonetic Annotation
It consists of labeling the various components of natural speech, such as intonation, stress elements, and pauses.
Part-of-Speech Tagging
This is where different function words are annotated.
These may be combined with other types of annotation like images, audio, and video whenever necessary to create fully-fledged AIs that can manage all types of data inputs. Creating such an AI requires the experience and capability of a competent data annotation company for the best results.
There are multiple steps applicable to text annotation, as it is a complex and arduous undertaking. The volume and variety of your data, the scale of the operation, schedule, budget, number, and type of applications the AI will be used for, and other related factors influence the number of steps and operations. Some steps are common across text annotation for every type of industry, which you can find listed below.
Data Preparation
Data preparation is an essential step in the text annotation process. It involves cleaning and preprocessing the text data to make it suitable for annotation. This step can include tasks such as:
Removing irrelevant or sensitive information from the text data
Removing special characters, numbers, and symbols that can interfere with the annotation process
Splitting text data into sentences or paragraphs to make it easier to annotate
Converting text data into a format that can be easily annotated, such as plain text or XML
Removing duplicates to eliminate redundancy and improve efficiency.
This is the stage where the annotation process occurs. This can be done using a variety of tools and techniques, such as annotation software or online platforms. The above-listed types of annotation types are considered, and the required ones are chosen based on the application the AI model will be used.
Evaluation
After the annotation process is complete, it is important to evaluate the quality of the annotations. This can be done by comparing the annotations to a set of pre-annotated text data, known as a gold standard. The evaluation process can also be done using metrics such as accuracy, precision, and recall. These metrics can help to identify any errors or inconsistencies in the annotations and ensure that they are of high quality.
Project Management
Annotation project management is the process of keeping track of the progress of the annotation project, including the number of annotated examples, the number of annotators, their performance, and the cost of the project. This step can include tasks such as:
Setting up a project plan and timeline
Assigning roles and responsibilities to annotators
Monitoring the progress of the annotation project
Identifying and addressing any issues or delays
Quality Control
Quality control is an essential step in the annotation process as it ensures that the annotations are consistent and accurate. This can be done by having multiple annotators label the same data and comparing their annotations or by using automated quality control tools.
This step may need to be repeated a few times to double-check the accuracy of the annotation process, especially if changes have been made during the QA cycle. Also, the quality check parameters and approach must be revised periodically to keep up with the changes in the field of annotation, market conditions, operational protocol alterations, business objectives, and other influential factors.
The practice of favoring the use of AI to make sense of textual data present in an enterprise’s data warehouse is evolving. With the application of excellent text annotation services, your company can gain further momentum in the race to extract maximum value from enterprise text data. Following the steps mentioned here will aid your company in becoming future-ready and establishing a strong market standing against tough competition. (SJ/GP)